I’m excited to welcome Wallaroo – the enterprise platform for production ML and AI – to the M12 portfolio, as lead of their Series A round.
One of my favorite aspects of the diligence process is speaking with a startup’s customers. When a customer is visibly excited to speak about a company’s product during a reference call, it’s always a good sign. But when this happens with every customer, you know that a startup is on to something great. This infectious evangelism is exactly what we felt firsthand when talking to users of Wallaroo. Wallaroo customers consistently shared that the tool made their teams more efficient and more effective at creating business value. This kind of enthusiasm is a testament to the customer centricity and prioritization of the user experience that drives the team at Wallaroo.
MLOps for More Efficient, More Effective Machine Learning
Wallaroo falls within the emerging space of Machine Learning Operations, or “MLOps.” MLOps applies DevOps principles such as continuous integration, delivery, and deployment, to increase the efficiency and effectiveness of machine learning workflow, and to enable enterprises to more easily build and scale machine learning models. Within the MLOps value chain, Wallaroo specifically solves the problem of enabling enterprises to take their machine learning models and seamlessly deploy these models into production at scale, a critical step in the MLOps life cycle rife with costly challenges, failure, and error.
Making an ML model run in the production environment at scale is very challenging, but it’s not sufficient to generate ROI. The realization of actual business value by deploying ML models in an efficient, iterative way to continuously improve results is a proposition fraught with challenges that still don’t have solutions, especially given the emerging and nascent nature of the machine learning operations field. Given these complexities and technical difficulties inherent in machine learning modelling operations/workflow, it is no surprise that enterprises face a great deal of frustration and failure in their AI and ML model deployment efforts. For instance, an estimated 50-60% of companies of all sizes developing machine learning or artificial intelligence have never deployed a single model they’ve spent time developing (and 90% don’t see any meaningful ROI in the end), costing global enterprises billions of dollars each year.
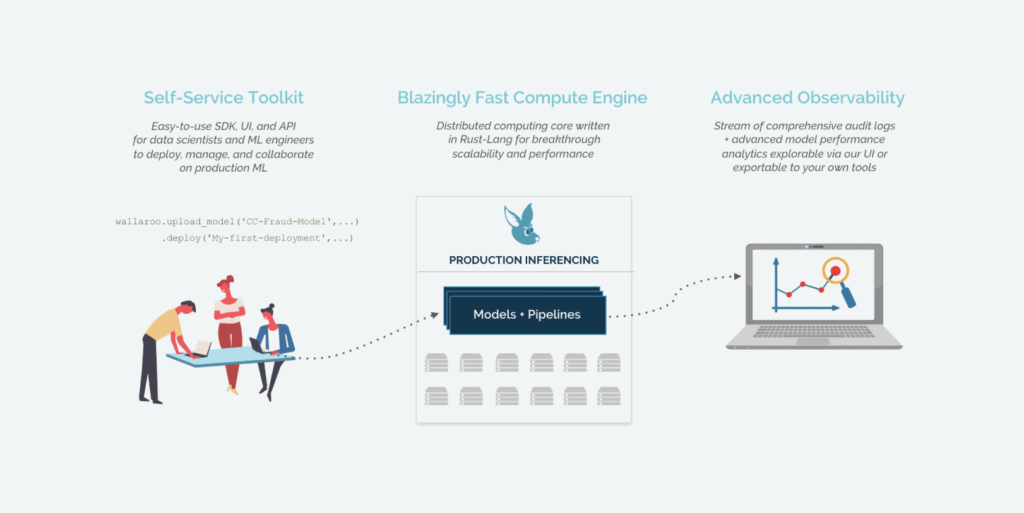
Machine Learning At Scale
Wallaroo’s product solves for this challenging bottleneck. The company offers a platform that serves as a central way to deploy AI, regardless of what ML platform is used to train the models and where the model gets deployed. The core of the Wallaroo solution is a platform built atop a distributed compute engine that via an SDK/API enables a data scientist or ML engineer building a model in a Jupyter notebook to scale to live commercial deployment with just two lines of python code. Wallaroo can “partner” or “integrate” with basically any solution in the MLOps ecosystem without any additional work given the SDK interoperability at any and all stages of the ML Pipeline. What’s more, Wallaroo builds observability and model insights into the core platform, necessary for compliance but also for ML teams to identify reasons for model underperformance so they can adapt.
The company has made a multi-year investment in a Rust based distributed compute engine which is at the heart of their ability to abstract effort to scale up and out models in production environments.
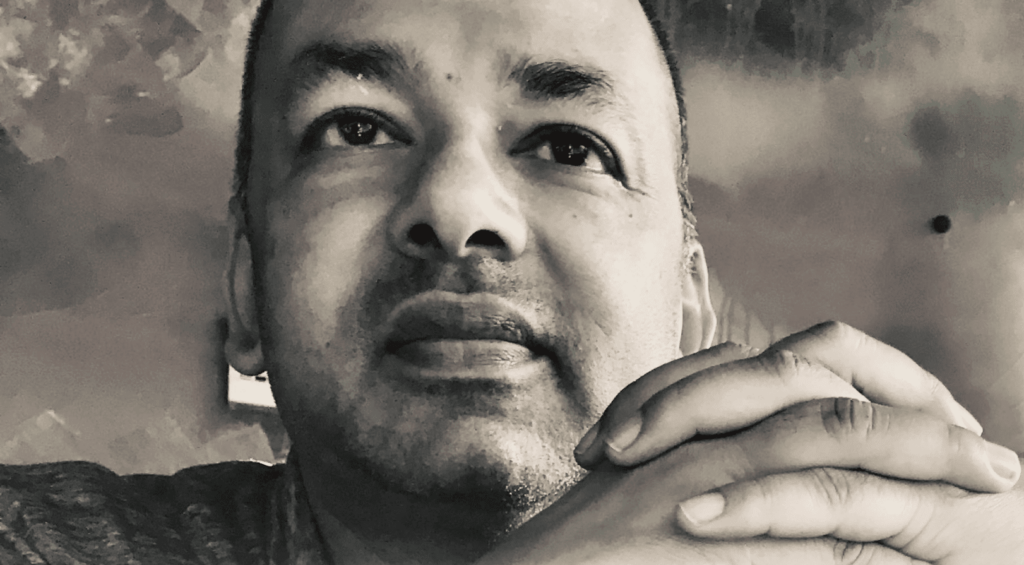